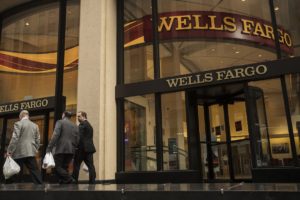
Wells Fargo Auto is looking beyond credit decisioning models for machine learning applications. Specifically, the company employed “sentiment analysis” — an automated process of understanding opinions from written or spoken language — for collections.
“One thing I was surprised to see is that through natural language recognition and sentiment analysis, it was very easy to predict customers’ thoughts about their loans and the company they are dealing with,” said Adem Yilmaz, senior vice president of dealer services compliance and operational risk, at the American Financial Services Association’s Vehicle Finance Conference last month. “If you identify that a customer has a negative view of your company and the collector, and you get a preventive action with that customer, you will probably be much better solving the issue with that customer than potentially making a lot of calls and trying to strike a deal.”
Yilmaz, who started to build out his team six months ago with the goal of enhancing the auto unit’s analytics, encouraged attendees to consider machine learning to analyze operational procedures. “Machine learning looks at the process itself to determine what are the big issues and what are the deficiencies,” then recommends certain changes, he said.
The applications for machine learning are wide-reaching, he noted. “Think of every activity that a human is doing,” he said. “Don’t just think of using it for credit decisioning or forecasting types of models. Think a little bit bigger.”